AI-Integrated Filtration System For Water Purification Using Algae And Bacteria-Based Filter
- JYP Admin
- Mar 30
- 5 min read
Author: Talin Hamdy
Abstract
Water purification represents a growing global concern, and traditional filtration methods often aren't effective enough in water filtration. Pollutants build up in the filters, leads to increase in fluid resistance and pressure drops, as described by Hagen-Poiseuille’s law, and this hinders the movement of water through pipes. Over time, this obstruction reduces efficiency and increases maintenance costs. This study introduces an effective AI-driven filtration system that can be installed within pipelines to treat pollutants and adjust filtration efficiency. The system combines Azolla algae, Bacillus subtilis, and Pseudomonas putida bacteria, which naturally break down contaminants. Bacteria stick to the surface of the cellulose membrane, increasing its ability to filter the water. At the same time, AI regulates the filter’s pore size based on sensor feedback, using a solenoid valve, and these sensors are connected to a website to clarify the results for users. The experimental results detected a great difference in reduction of turbidity, organic pollutants, and nutrient levels, increasing the system’s effectiveness. Additionally, the research highlights the role of physics principles, especially fluid dynamics and diffusion, in optimizing filtration that are considered in system design.
Introduction
Access to clean water is essential for human health and environmental sustainability, but traditional water filtration methods often cannot adapt to fluctuating pollution levels. Biological filtration techniques have long been recognized for their ability to degrade contaminants by leveraging the metabolic activities of bacteria and algae, which can lower overall efficiency. To solve this, an Azolla algae, Bacillus subtilis, and Pseudomonas putida bacteria are used in a system designed, where each plays a specific role in water purification. For example, the algae absorb excess nutrients like phosphorus and nitrogen, while the bacteria break down organic pollutants.
Also, using a mix of microorganisms helps pollutants spread more evenly in the water, improving diffusion rates and speeding up the purification process. The bacteria attach to a cellulose membrane, increasing the surface area for filtration while keeping flow resistance low, so water moves through efficiently without clogging.
Research shows that adding Bacillus subtilis to wastewater left after filtration helps improve denitrification and boosts butyrate production during glucose fermentation (Rahimi et al., 2020), other studies highlight the key role of algae in wastewater treatment by absorbing carbon, phosphorus, and nitrogen in an effective way (Bhatt et al., 2020). Building on these researches, this research integrates bioaugmentation with an AI-driven system to create a smarter and more efficient water filtration system.
Aim of Study
The goal of this research is to integrate an AI system that has the ability to adjust dynamically to varying pollution levels with biological filtration methods, aiming to improve water purification efficiency within pipelines. Physics-based filtration mechanisms, such as the movement of water through porous materials and the interactions between pollutants and biological media, play a central role in this research.
Materials and Methods
The filtration system uses biological layers of Azolla algae, Bacillus subtilis, and Pseudomonas putida bacteria sticked on cellulose membrane, this combination work together to break down contaminants. Integrated AI detects pollutant concentrations and automatically adjusts the filter’s pore size to enhance purification. Understanding the influence of hydrodynamic forces, diffusion, and adsorption mechanisms is crucial in optimizing filtration performance.

Preparation of Bacteria and Algae Filter: The Bacillus subtilis strain (EMCC 1009, isolated from natto food, Japan) and Pseudomonas putida were obtained from Cairo MIRCEN (Faculty of Agriculture, Ain Shams University, Cairo, Egypt). The two bacterial strains were cultured separately before being introduced into the filtration system. For Bacillus subtilis, DSM agar plates (composition: peptone, 5 g; meat extract, 5 g; agar, 15 g per liter; pH 7.0) were prepared, and the bacteria were incubated at 30 °C for 48 hours to develop active colonies, Pseudomonas putida was cultured on nutrient agar plates under similar conditions. The Media of both strains were prepared in 500 mL flasks, then sterilized at 121 °C for 15 minutes, and cooled before inoculation. The bacteria were separately incubated in their respective media before being introduced into the system. The Azolla algae were co-cultured with Bacillus subtilis and Pseudomonas putida at 18 °C with gentle shaking. After 21 hours, the mixture was left to dry and then incorporated into the filtration experiment.

Media Preparation:


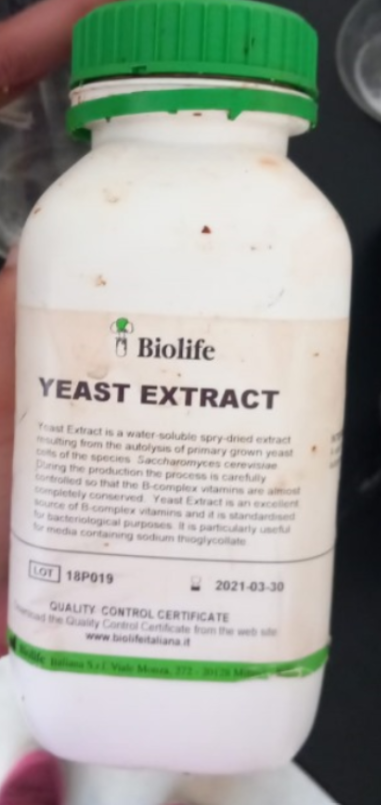



Sensor Integration for System Monitoring: Multiple sensors were integrated to measure key water quality parameters:
Turbidity Sensor checks the amount of particles floating in the water.
pH Sensor monitors changes in acidity and alkalinity.
TDS Sensor measures dissolved organic and inorganic substances.
Dissolved Oxygen Sensor measures oxygen levels needed for microbial activity in water.
Ammonia and Nitrate Sensors detect nutrient pollutants affecting filtration efficiency.
Water Flow Sensor controls the passage of water and ensures stable filtration rates.
AI-Based Pollutant Detection Sensor analyzes pollutant concentration and connects the data taken with the website.
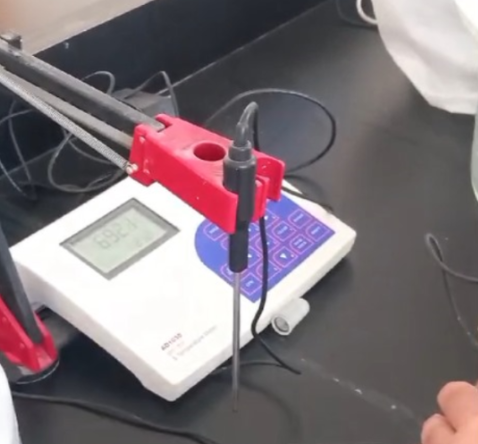
![Turbidity sensor used to control water quality [Source: https://www.libelium.com]](https://static.wixstatic.com/media/3f045e_731f78b4f6cf4136b60cead77d9ee7d9~mv2.png/v1/fill/w_427,h_412,al_c,q_85,enc_avif,quality_auto/3f045e_731f78b4f6cf4136b60cead77d9ee7d9~mv2.png)
![Dissolved oxygen sensor [Source: https://disen-sensor.com/top-9-water-quality-sensors-for-wastewater-treatments/]](https://static.wixstatic.com/media/3f045e_d4828be1b6784475a174930bb4a1745d~mv2.png/v1/fill/w_427,h_423,al_c,q_85,enc_avif,quality_auto/3f045e_d4828be1b6784475a174930bb4a1745d~mv2.png)
Experimental Setup and Results
To test the filtration system, two bottles connected by a small opening were used to act as the pipeline, the filtration medium placed between them. Water was passed in the pipeline through the Bacillus subtilis bacteria membrane, the Azolla algae membrane, and finally passed by the Pseudomonas putida bacteria. Sensors detected pollutant levels before and after filtration for over 49 hours. The experiment was designed based on diffusion and fluid resistance in porous media, which helped in adjusting the flow rate for better system.
Results:

Conclusion
This AI-integrated filtration system offers a promising and adaptive solution for water purification. By leveraging real-time sensor feedback, it efficiently regulates filtration properties, resulting in significant water quality improvements. The study underscores the importance of integrating microbial interactions, fluid dynamics, and AI-driven optimization in modern water treatment technologies. Future work will focus on refining AI algorithms, extending field tests, and exploring large-scale applications to assess the system’s long-term effectiveness.
References
[1] Zhao, Y., Lian, H., Tian, C., Li, H., Xu, W., Phuntsho, S., & Shih, K. "Surface Water Treatment Benefits from the Presence of Algae: Influence of Algae on the Coagulation Behavior of Polytitanium Chloride." Frontiers of Environmental Science & Engineering, vol. 15, article 58, 2021, doi:10.1007/s11783-020-1350-x.
[2] Li, L., Rong, S., Wang, R., & Yu, S. (2021). Recent advances in artificial intelligence and machine learning for nonlinear relationship analysis and process control in drinking water treatment: A review. Chemical Engineering Journal, 405, 126673.
[3] Mujtaba, G., Rizwan, M., & Lee, K. (2017). Removal of nutrients and COD from wastewater using symbiotic co-culture of bacterium Pseudomonas putida and immobilized microalga Chlorella vulgaris. Journal of Industrial and Engineering Chemistry, 49, 145-151.
[4] Zhu, Z., Wu, Y., Fang, X., Zhong, R., Gong, H., & Yan, M. (2024). Bacillus subtilis, a promising bacterial candidate for trapping nanoplastics during water treatment. Journal of Hazardous Materials, Article 136679.
[5] Zhang, X., Lei, Z., Ge, S., Ji, B., & Zhang, B. (2023). "Editorial: Algae and Microalgae-Bacteria Based Technology for Sustainable Wastewater Treatment." Frontiers in Microbiology, 14, 1263955. doi:10.3389/fmicb.2023.1263955.
[6] Emamgholizadeh, S., Kashi, H., Marofpoor, I., & Zalaghi, E. (2014). "Prediction of Water Quality Parameters of Karoon River (Iran) by Artificial Intelligence-Based Models." International Journal of Environmental Science and Technology, 11(3), 645–656. doi:10.1007/s13762-013-0241-1.
[7] Rawat, I., Kumar, R. R., Mutanda, T., & Bux, F. (2011). "Dual role of microalgae: Phycoremediation of domestic wastewater and biomass production for sustainable biofuels production." Applied Energy, 88(10), 3411-3424. doi:10.1016/j.apenergy.2010.11.025.
Comentarios